IntrinsicDiffusion: Joint Intrinsic Layers from Latent Diffusion Models
Jan 1, 2024·,,,,,,,,·
0 min read
Jundan Luo
Duygu Ceylan
Jae Shin Yoon
Nanxuan Zhao
Julien Philip
Anna Frühstück
Wenbin Li
Christian Richardt
Tuanfeng Y. Wang
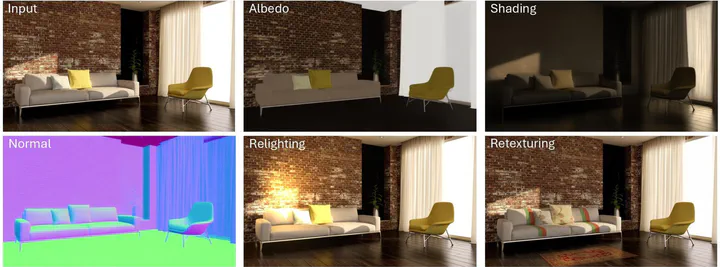
Abstract
Reasoning about the intrinsic properties of an image, such as albedo, illumination, and surface geometry, is a long-standing problem with many applications in image editing and compositing. Existing solutions to this ill-posed problem either heavily rely on manually designed priors or learn priors from limited datasets that lack diversity. Hence, they fall short in generalizing to in-the-wild test scenarios. In this paper, we show that a large-scale text-to-image generation model trained on a massive amount of visual data can implicitly learn intrinsic image priors. In particular, we introduce a novel conditioning mechanism built on top of a pre-trained foundational image generation model to jointly predict multiple intrinsic modalities from an input image. We demonstrate that predicting different modalities in a collaborative manner improves the overall quality. This design also enables mixing datasets with annotations of only a subset of the modalities during training, contributing to the generalizability of our approach. Our method achieves state-of-the-art performance in intrinsic image decomposition, both qualitatively and quantitatively. We also demonstrate downstream image editing applications, such as relighting and retexturing.
Type
Publication
SIGGRAPH 2024